Forecasting Social Navigation in Crowded Complex Scenes
Year: 2015
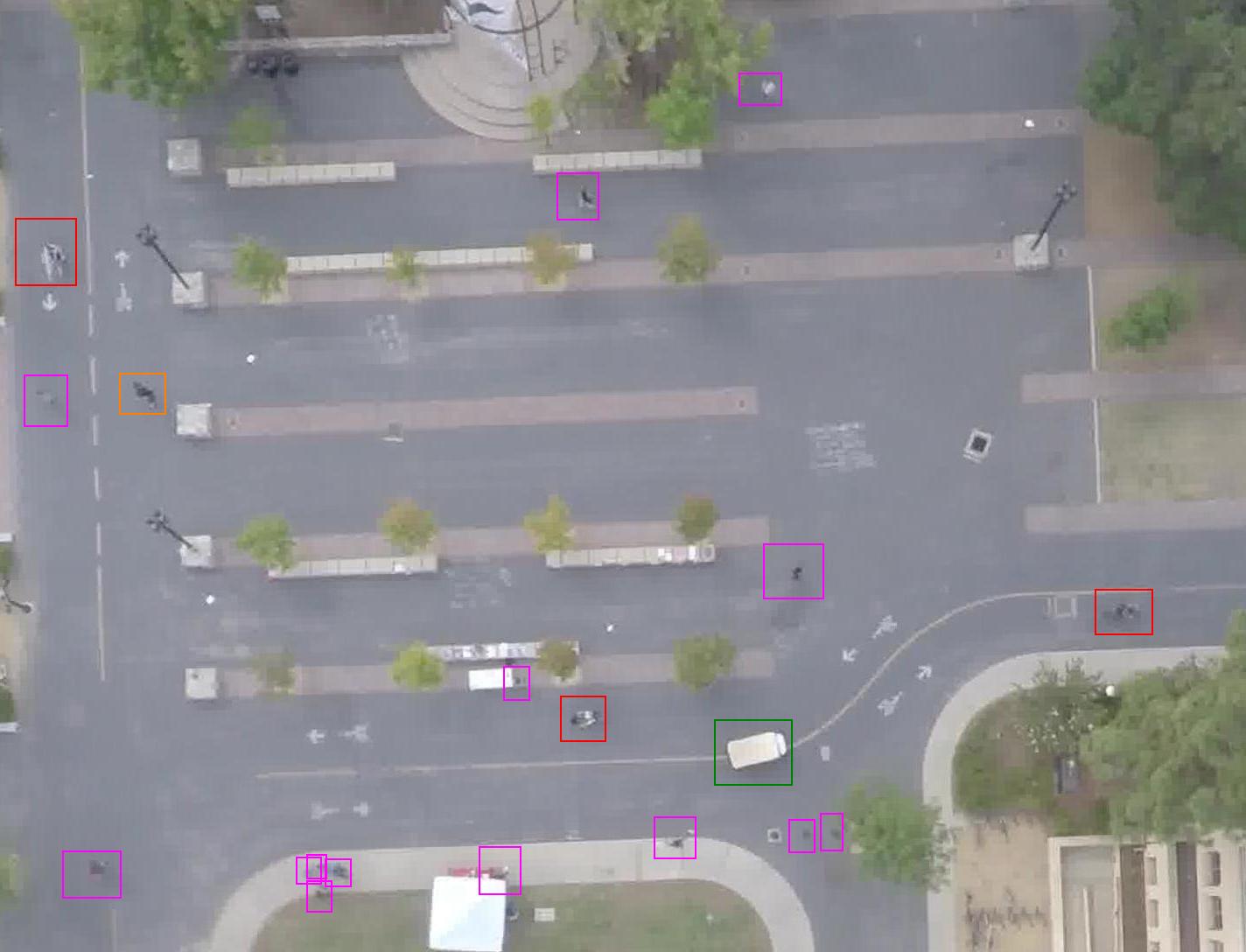
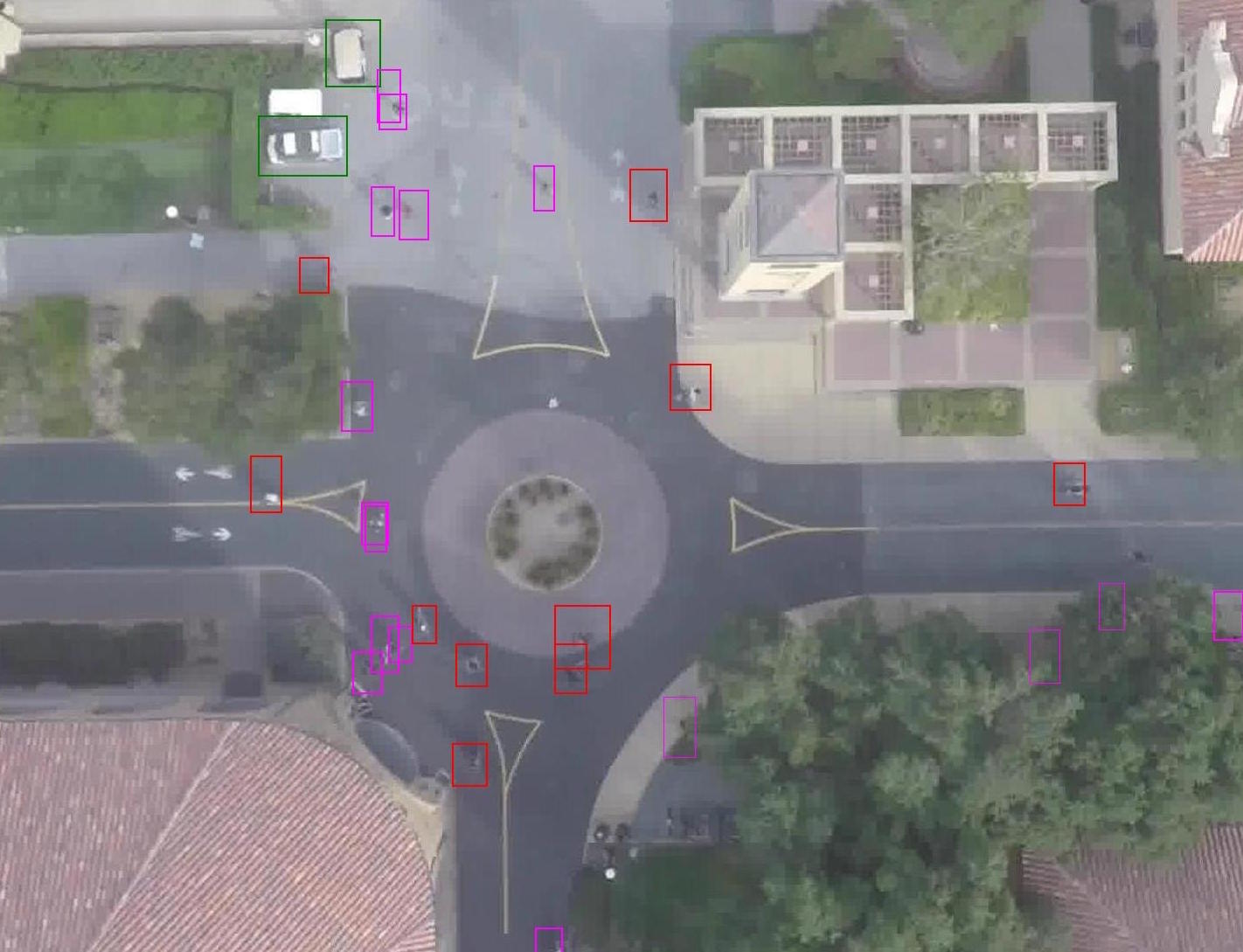
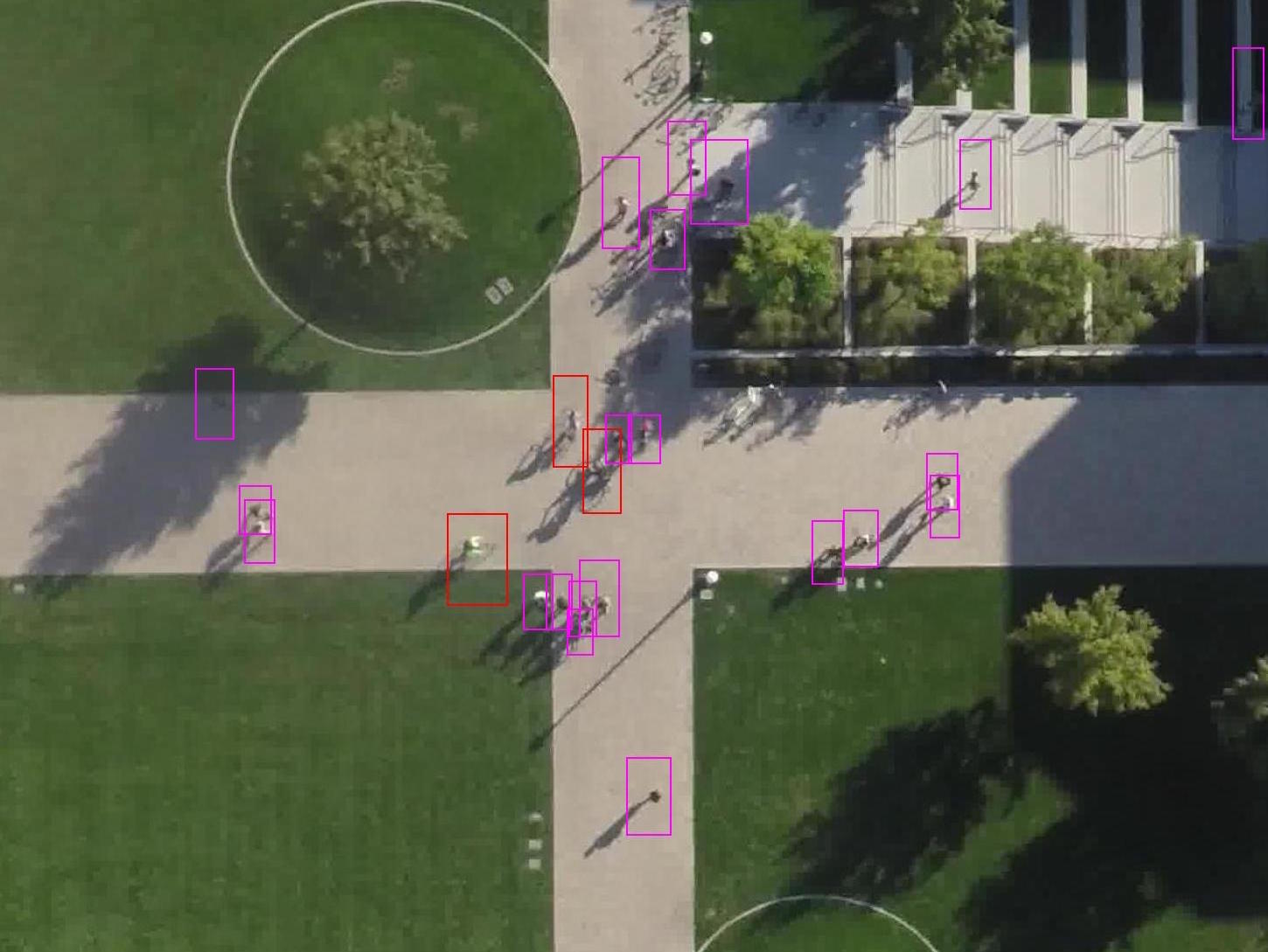
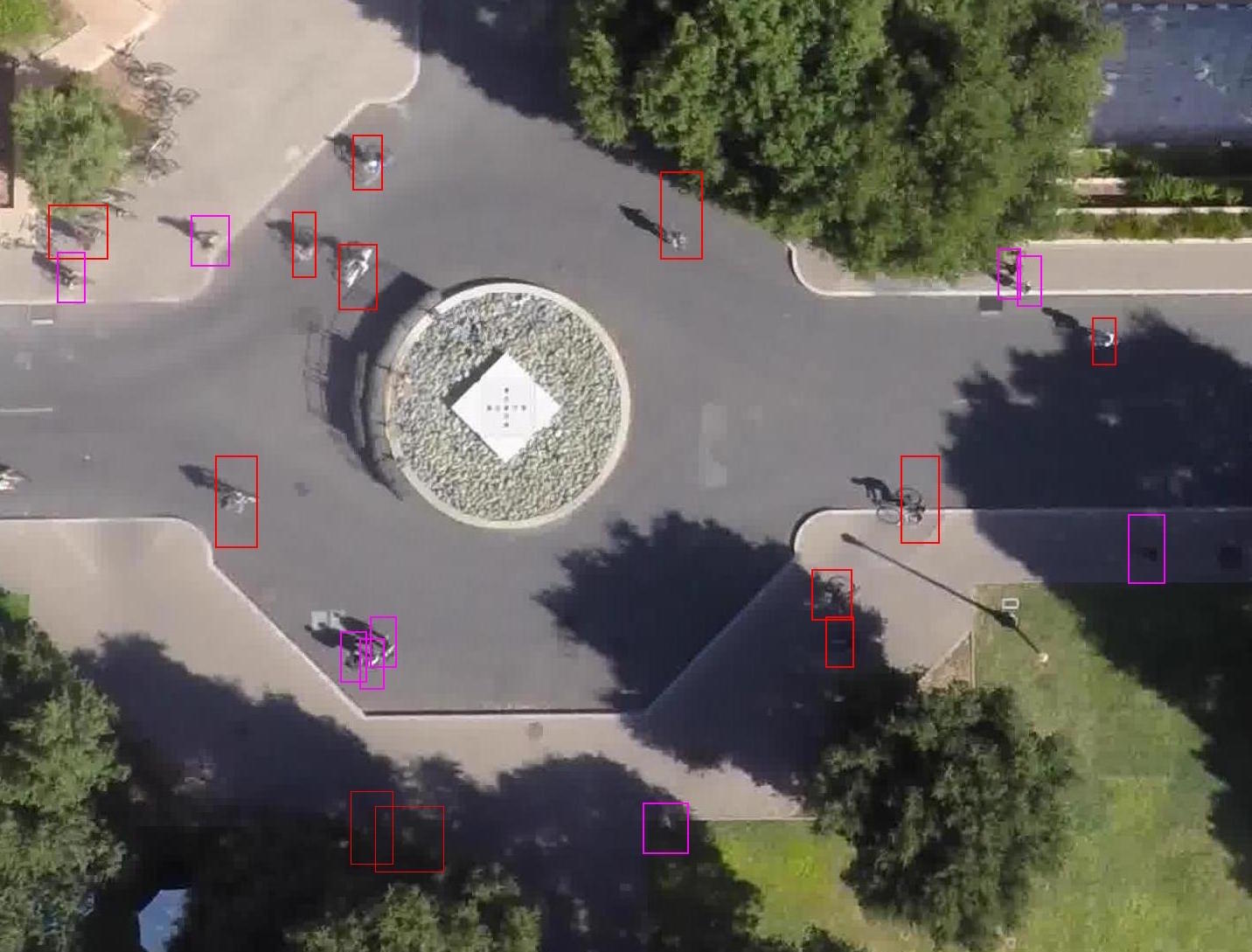
AuthorsPermalink
Alexandre Robicquet, Alexandre Alahi, Amir Sadeghian, Bryan Anenberg, John Doherty, Eli Wu, and Silvio Savarese
AbstractPermalink
When humans navigate a crowed space such as a university campus or the sidewalks of a busy street, they follow common sense rules based on social etiquette. In this paper, we argue that in order to enable the design of new algorithms that can take fully advantage of these rules to better solve tasks such as target tracking or trajectory forecasting, we need to have access to better data in the first place. To that end, we contribute the very first large scale dataset (to the best of our knowledge) that collects images and videos of various types of targets (not just pedestrians, but also bikers, skateboarders, cars, buses, golf carts) that navigate in a real world outdoor environment such as a university campus. We present an extensive evaluation where different methods for trajectory forecasting are evaluated and compared. Moreover, we present a new algorithm for trajectory prediction that exploits the complexity of our new dataset and allows to: i) incorporate inter-class interactions into trajectory prediction models (e.g, pedestrian vs bike) as opposed to just intra-class interactions (e.g., pedestrian vs pedestrian); ii) model the degree to which the social forces are regulating an interaction. We call the latter ”social sensitivity” and it captures the sensitivity to which a target is responding to a certain interaction. An extensive experimental evaluation demonstrates the effectiveness of our novel approach.
ResourcesPermalink
TechnologiesPermalink
- Python
- Javascript
- Amazon Mechanical Turk